In 2012, the Harvard Business Review called Data Scientist “the sexiest profession of the 21st century,” and five years later, IBM predicted that by 2020, there will be 2,720,000 open positions for professionals who collect, analyze, and interpret complex digital data. Over time, IT companies in the country have also started looking for such experts, and today H512.com’s job board has over 170 open Data Science (DS) positions (mostly for Sofia and fully remote), giving the right candidates the opportunity to work as “information anatomists” in their own country.
Applications, roles and opportunities – the irresistible sides of Data Science
Big Data Is Big Business has become the mantra of the century. As a result of rapid technological development, companies have access to vast amounts of information, and by 2025 we will generate more than 181 ZB of data (181 with 21 zeros!). This makes the DS role, to say the least, all-encompassing:”
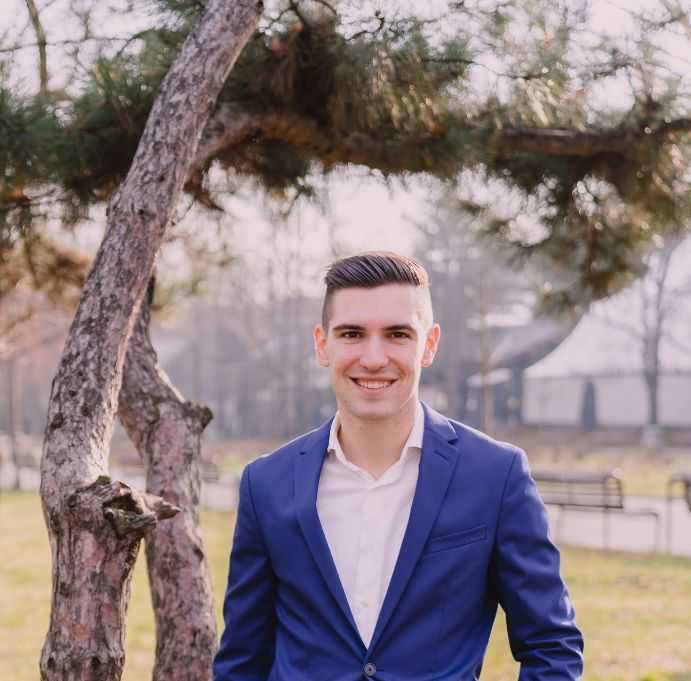
“The data scientist profession finds its place in almost every field – from logistics and transportation to education and ecology. Artificial intelligence and statistics today are actively entering even the field of art. Through the innovative Stable Diffusion model, for example, anyone can now easily materialize their ideas into an automatically generated picture – something few imagined would be possible,” says Georgi Gulyashki, Data Scientist at Transmetrics, and adds that DS will find wider application where there is a shortage of manpower and resources, such as telemedicine.
However, the sectors that are currently using DS most actively include e-commerce, automotive and aerospace, healthcare, pharma, finance, telecoms, online betting and many others, according to the observations of Georgi Pamukov, Data Management & Data Science Practice Lead at Adastra: “They are more data dependent, invest in R&D, apply innovations, also use unstructured data, rely on real-time customer-facing models beyond standard ones.”
The entry of DS into more and more business and social areas will change both the way work is done and the roles themselves: “Until recently, a single team was responsible and capable of performing all analytical tasks end-to-end. With growing volumes of information, new methods and technologies have emerged and now distinct components of the so-called pipeline have emerged, each taken on by a separate group of specialists. New roles are emerging and these are often related to proficiency in one core technology or deep knowledge in just one of the data extraction, initial processing, modelling and visualisation steps. This calls for a narrower specialization of any modern successful data scientist,” says Georgi Gulyashki.
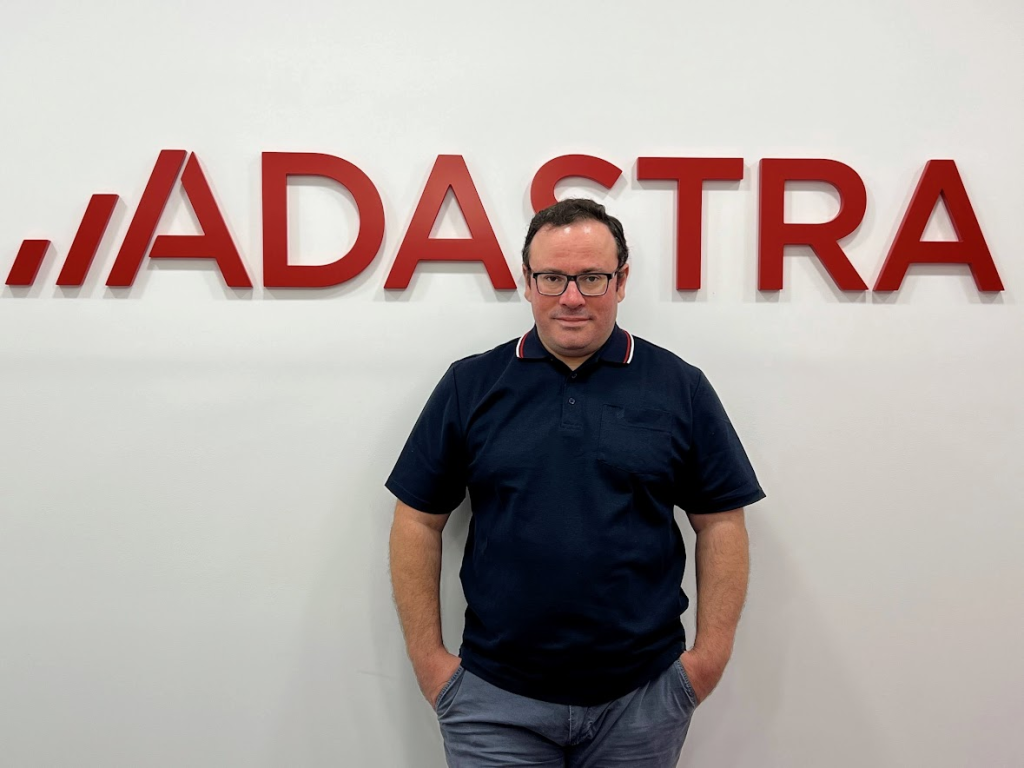
Georgi Pamukov gives more details about these specializations. One of the roles with great potential is Deep Learning (DL) Engineer as a result of data science trends and these specialists will be in demand because companies are now looking beyond structured and tabular data and working on a variety of applications based on photo, text, audio and other data.
Other ‘hot’ positions include Edge DL/AI and MLOps/DLOps Engineers: ‘These are just some of the roles – the potential is literally everywhere! With the rise of AI, the demand for various specialists around ethics and bias is likely to increase, and as quantum computing develops, relevant equivalents on the data structures side will start to emerge. Concepts such as federated learning are also evolving and will likely lead to demand for relevant specialists. One thing is certain – interesting times are ahead.”
According to Danislav Zhelyazkov, Data Scientist at Ocado Technology, wide-ranging models will be used in a variety of scenarios and more Generative AI engineers will be in demand, “Quite often Data Science is used for data mining and image analysis. But interestingly, it can also be applied in many other use cases such as optimizations, operations research, any type of path finding, task planning and allocation, demand forecasting and other types of predictions. As the saying goes, “The sky is the limit.”
The Herculean demands of the profession
The road to DS is paved with good intentions and thousands of opportunities, but it is also one of the thorniest. As Adastra‘s Georgi Pamukov says, it’s a multifaceted discipline and the career steps are different for everyone. Personally, he has a maths and economics and statistics background, as well as real-world experience as an analyst from when DS didn’t exist as a concept. However, to become a practitioner he lacks the necessary programming skills (SQL & ETL, R, Python, etc). He invested time and effort to acquire them, and to learn proper methodology, terminology, project organization, initial ML skills, etc., he went through Johns Hopkins University’s DS specialization. This was followed by gaining hands-on experience and knowledge in ML, to which Kaggle was a major contributor. It was only then that he secured his first “real” DS position as an ML engineer: “This was just the beginning of a path that subsequently took me through Data Scientist, Senior Data Scientist and Data Science Lead positions in various companies and industries. It is important to understand that a career in this field involves a huge and constant investment of effort and time.”
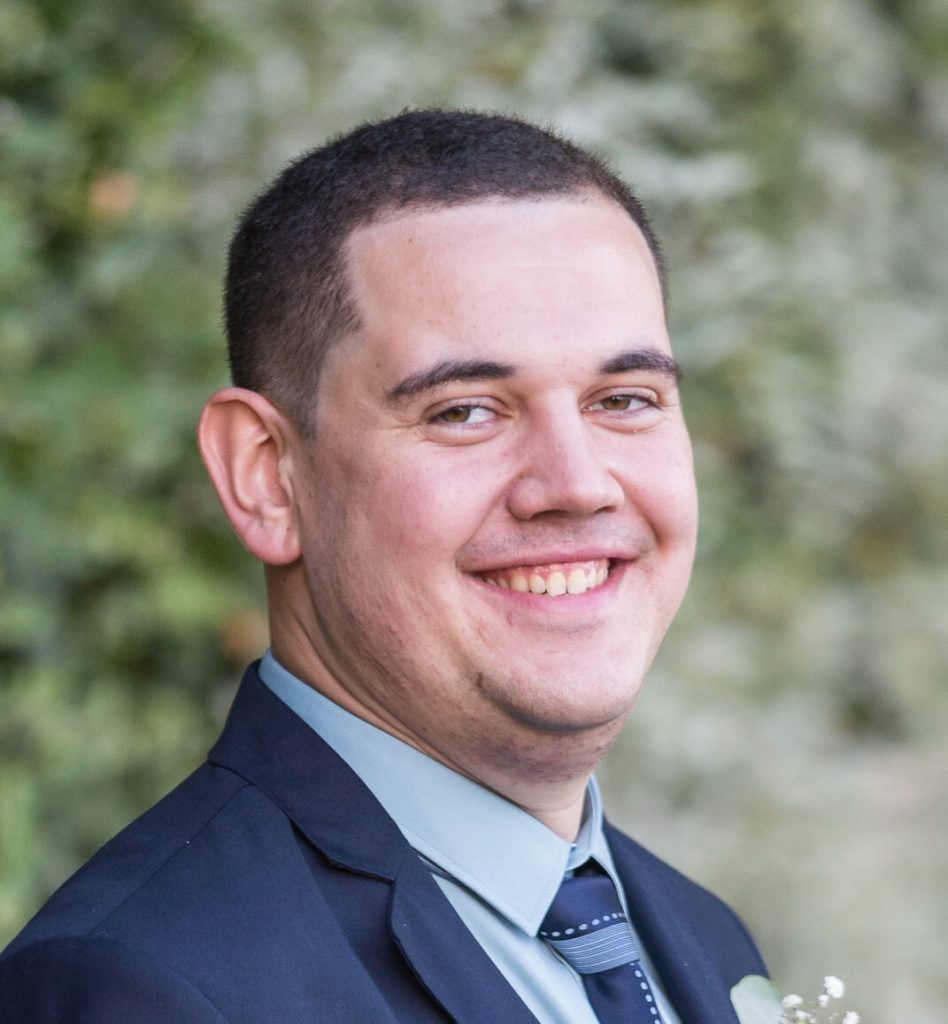
The starting point of Danislav Zhelyazkov’s career at Ocado Technology is diametrically opposed, because he started as a programmer – a Bachelor’s degree in Computer Science, a Master’s degree in Artificial Intelligence, a job as a software engineer and after a few courses became a Data Scientist. The important thing is to be driven and know what you want. The field is quite dynamic and the standard and best technologies are constantly evolving.”
Regardless of the direction in which a prospective DS professional heads, there is a mandatory set of skills to acquire and develop in order to succeed. Some of these include:
- A solid theoretical background in mathematics and statistics, including what is known as cognitive biases: “It is no coincidence that the word science is in the verbiage. Every ML model has a specific mathematical formulation and understanding it is key for its correct application and for the interpretation of the results,” says Georgi Gulyashki from Transmetrics.
- Highly developed analytical thought and a deep understanding of the field and industry in which he works (domain knowledge).
- Impeccable computer skills – as Georgi Pamukov’s story illustrates, these can be acquired later if they step on mathematics and logic.
- Developed methodological, scientific and organisational skills.
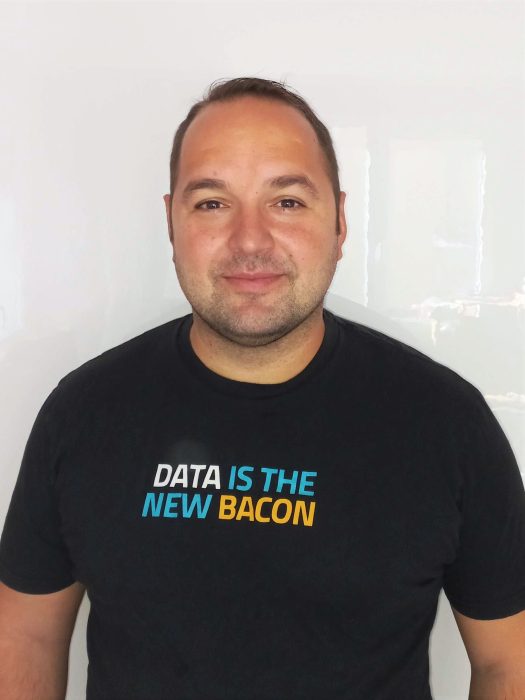
- Data skills, visualizations, ML/DL: “A young professional usually has theoretical knowledge about different algorithms, but lacks the practical work on data exploration and problems. With time and experience, he is expected to handle the usual problems with ease – data quality and lack of data, defining and confirming or rejecting hypotheses, visualizing data in an appropriate form,” explains Milen Chechev, Head of Data Science at Fourth.
- Very good teamwork skills.
- Language, communication and presentation skills are a must – be able to tell data stories in ‘plain’ language and argue them intelligibly to IT and business audiences.
- Curiosity, attitude, willingness and discipline to continuously build on knowledge by reading scientific literature and detailed research into the latest trends.
And more. DS is increasingly collaborating with the humanities and this “spillover” means only one thing – filling knowledge gaps on both sides: “Nowadays, sociology and psychology rely heavily on Data Science. This is clearly reflected in the curricula of the world’s leading universities – in the Experimental Psychology degree at Oxford, for example, the only compulsory subject is Probability Theory and Statistics. In this line of thought, people who want to develop in the social sciences should inevitably emphasise the study of mathematics and statistics. After all, these exact sciences only serve as a means for well-founded reasoning. Their application in the work of social scientists, psychologists and economists requires good collaboration between the different fields to provide sufficient context and knowledge in the formation of hypotheses, scientific method and experiments,” says Georgi Gulyashki of Transmetrics.
The dizzying growth of data volumes is not only leading to the emergence of new tech roles, specialisations and more active communication between individual scientific fields, but in places is also starting to change the job market in unexpected ways. Most recently, news broke that data giants Scale AI and Appen are looking to hire professional poets and writers with familiar and exotic languages to improve the literary qualities of their generative writing tools. Apparently no matter how fast technology advances, there is an element that (for now?) remains the preserve of man alone.
The place of creativity in datasets
Today, everyone talks about the shortage of IT staff, and in the context of DS, this shortage includes a shortage of data ingenuity, or so-called creativity. To become really good, a professional must have the ability to think like a poet (in fact, the term was first used formally in the 17th century by the Polish poet Matej Sarběwski), or outside the familiar technological box, because that is exactly what companies are looking for to be competitive in today’s business environment. What exactly is creativity in the context of data science?
For Georgi Gulyashki of Transmetrics, it’s a quality that helps experts extract the most useful data from the information fog: “In practice, data is often ‘dirty’ and insufficient. This is exactly why one of the most challenging tasks of the profession is to form a quality data set that contains the most valuable information possible, fit for modelling. This is where creativity plays a huge role – with the right statistical approach, being able to create additional useful variables based on those that already exist. Extracting and detecting missing values and large deviations from the available information is often a job without predefined steps, and sometimes transforming the data from its original form can be compared to squeezing water from a stone.” Once the core of the problem has been identified by way of creativity, the specialist will have no problem recognizing its analogue in another domain.
Danislav Zhelyazkov of Ocado Technology is of the same opinion: “There is a lot of data. The problem is that they are in raw form and we cannot use them directly. It is crucial to select heterogeneous data, to make sure it is diverse enough and equally distributed to the case in which we will use it. If we don’t have enough, we can try synthesizing them with some type of Data Augmentation. For this to happen, however, we more or less need to understand the data we have. This is where statistics comes in. There are also many pre-analysis tools available that quickly give us a general idea of them and offer some automatic initial processing. Many already well trained models are publicly available, we just need to check how well they work in our case and evaluate them with our data. If necessary, we can also adapt them further.”
According to Fourth‘s Milen Chechev, one of the most important skills in the profession is, when looking at data, to ask questions, make hypotheses and experiment to prove or disprove those hypotheses: “It’s a time-consuming process that requires a lot of ingenuity, logic and problem-solving skills, and different challenges may require combining different algorithms from machine learning and computer science.”
Finally, creativity is not only a key business differentiator but also one of the main criteria for whether a Data Scientist is a talent or just a performer: “Creativity is one of the qualities that is the watershed between good and mediocre professionals in any field, and it is becoming increasingly important in the GenAI era. While tedious and repetitive tasks will increasingly be entrusted to AI, understanding the problem and inventing the approach to solve them will remain with us humans. This is directly related to creativity and it is present everywhere in the Data Science domain,” says Georgi Pamukov from Adastra. He adds that creativity is the child of knowledge and motivation – the desire to solve a problem in the best possible way and in untrodden paths, and general data culture – the mastery of different approaches and multiple “outputs”, which allows to combine them when deciphering more problems and even inventing new methods.
Weak spots in the Data Science talent supply chain
The demand for DS experts exploded during the pandemic, when digital transformation turned on very fast speed. However, the shortage continues to be palpable. This is, on the one hand, due to the fact that the profession is relatively new on the Bulgarian market and it is difficult to find staff with relevant knowledge and experience. On the other hand, even if they are available, the challenges are related to their level of expertise: “A large part of the relevant programmes in most Bulgarian universities are focused on theoretical, sometimes not so useful knowledge. The practical aspect is not well covered and is often outdated. This is a highly dynamic discipline and universities are struggling to keep pace. In many cases Data Science is taught by people with no practical experience and no real understanding of the field, leading to sub-optimal results. The positive aspect is that candidates who graduate from university often have the necessary statistical background as well as some programming skills,” says Adastra‘s Georgi Pamukov. He adds that the difficulty for experienced programmers is finding more highly specialised DS profiles, and the recruitment process itself is exacerbated by the fact that unqualified candidates send CVs en masse for every vacant position, simply because the discipline is “hot”.
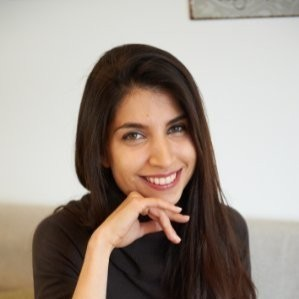
According to Styliana Kanjeva, Senior Recruiter at Fourth, the increasing demand for DS professionals is also affecting the recruitment process. At the very first interview they meet the team manager, who gives an in-depth view of the role. Then, at a technical interview, they get to know colleagues they would be working with directly. In this way, both parties understand more about each other and the ultimate goal is to be able to make as informed a decision as possible.” And with ready-made staff in short supply, the main source of new talent at Fourth remains the well-known recommendations of current employees – over 40% of experts are hired this way. Other sources for sourcing in-demand talent include the company’s internship program, regular internal sessions to develop knowledge in data teams, research activities, and more.
In fact, most of the IT companies follow the maxim “Create your own Data Science talent” instead of waiting for the market to provide it. Programmers at Ocado Technology, for example, have access to the resource-rich O’Reilly and DataCamp platforms to build on their knowledge: ‘A personal budget is provided to every colleague in the team, which we use for external courses, books and conferences, and Friday is the day to learn. We also have regular sessions to share knowledge and issues with other colleagues at team, department and global level. Hackathons are also regularly organised to unravel specific work cases, and sometimes individual teams run general and specialised courses for interested colleagues,” says Danislav Zhelyazkov.
We understand from Georgi Pamukov that Adastra has an internal DS program for beginners and advanced. The basic level covers the entire DS cycle and includes all the basic steps (problem definition, data extraction & transformation, exploratory data analysis, feature engineering/selection, reprocessing, ML/modelling, evaluation, etc.) to create a solid foundation for understanding the concepts, methodology and the right approach to solving problems through practical tasks. The level culminates with participation in a playground competition on the DS platform Kaggle and can be built upon with advanced training that includes specific topics such as advanced modelling & ML, DL, NLP, optimizations and MLOps. The much talked about GenAI is not missed as well as other topics that help to make sense out of data sets.
We live in a world of constant change and uncertainties, and today every organization has a long list of complex problems to be solved by talent with the necessary skills, motivation, and overall data culture. This requires big ideas that have long been based on the insights gleaned from data sets, and these ideas will not only benefit IT companies, but communities as a whole because only their accurate analysis and creative application will take us to the next level.